Case Study: Integrating AI into an Existing Workflow
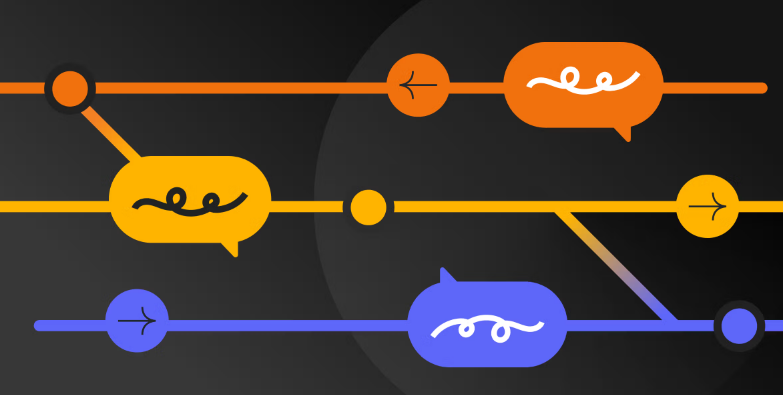
In September this year, we integrated AI to replace a task in our team’s workflow that had been a bottleneck since the launch of our company. In our work, we provide data and then summarize the data to be used in court cases and insurance claims settlements. Specifically, we mine social media and physical surveillance data to provide a picture of claimant or plaintiff’s lifestyles.
The actual summarization of the evidence was our single greatest pain point because it involved writing skills that are difficult to teach and also difficult to maintain uniformly across our production team. This kind of pain point is an ideal candidate for artificial intelligence to take over.
What we THOUGHT the solution would look like, and the ACTUAL solution were quite different. We assumed that we would create reports as usual, and then run AI to create the summary. However, the AI worked much better by moving it up the creation chain. The end result is that the AI would run a summary on each individual post and then we used AI to add all the individual post AI content together to create a summary.
Why?
When we ran AI on a full report, the AI became too interpretive and we lost control of the output. And, because our output is considered evidence, we needed more detail and pinpoint accuracy than the AI could provide when interpreting a larger mass of content.
Instead, by moving AI up to smaller interpretations, we could gain the speed we were looking for while maintaining the accuracy needed to be successful.
What is the learning for Insurance?
As AI becomes integral to insurance handling – underwriting and claims likely being the first to automate — the most important consideration is where to insert the technology. If it is tacked onto the end of a lengthy, data rich process, it can produce results that are “too interpreted” and it will be difficult for your teams to understand how or where to correct the output.
So why use AI at all?
As the labor market continues to tighten, it may not always be possible to hire perfectly-qualified candidates. By supplementing high-academic skills (writing/analysis/interpretation) with AI, the hybrid worker delivers better output than the worker alone.
Cost/Benefit Analysis
We were surprised to learn that our LLM (Large Language Model) partner was not very expensive, and the integration into our process was not that difficult from a technology perspective. The act of pushing out small amounts of content for AI analysis and then pushing back the results into a database does not require the heavy lift in skill or financing that we had anticipated.
Our team is using the AI, but it took them a while to adjust to the workflow change, where instead of focusing on the large final project, the focus on making sure all the small details were fully fleshed out prior to generating the large final report with technology. As the insurance industry moves into AI, the savings can be realized in efficiency and quality of output if the AI is inserted into the right place in workflow.